Neurological Institute Outcomes
Sleep Disorders
Multiple Sleep Latency Test
Machine Learning to Predict Multiple Sleep Latency Test Results with Polysomnography
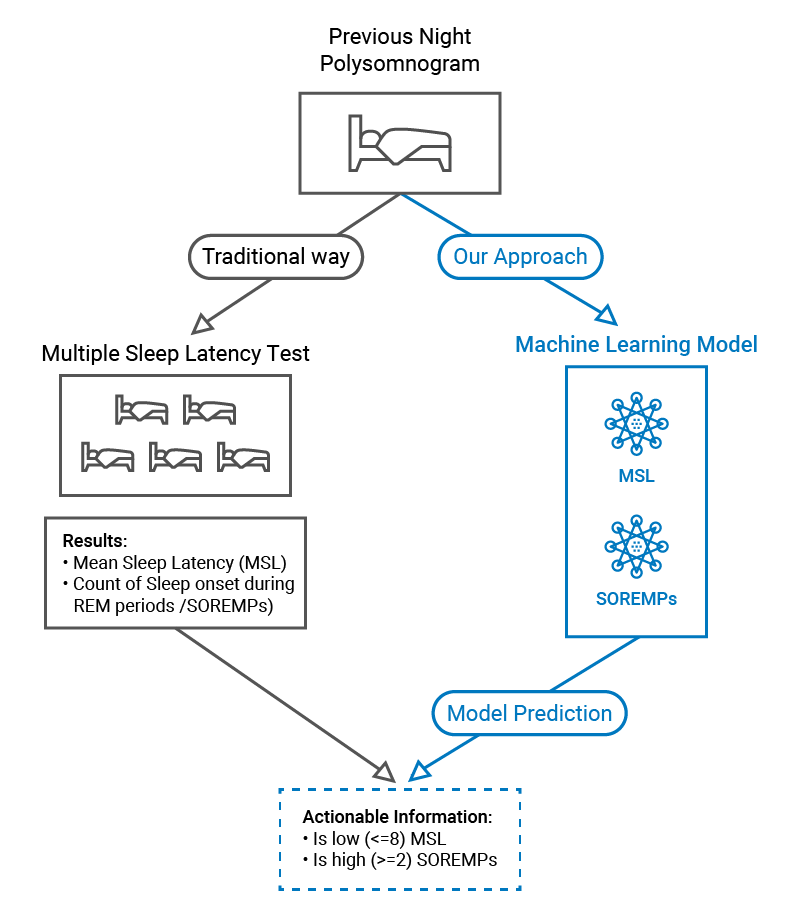
ESS=Epworth Sleepiness Scale, MSL=mean sleep latency, PSG=polysomnography, SOREMP=sleep onset REM periods.
Of 802 Multiple Sleep Latency Tests (MSLT) on 796 patients (75% female, age 34 ± 12.1 years, BMI 27.9 ± 7.6kg/m², ESS 13.9 ± 4.9) performed between 2012 and 2022, 343 (43%) showed mean sleep latency (MSL) < 8 minutes and 234 (29%) showed ≥ 2 sleep-onset REM periods (SOREMP) suggestive of pathological sleepiness. Five machine learning models (logistic regression, support vector machine, multilayer perception, random forest, and XGBoost) were trained to predict the presence of these two findings. Feature selection analysis was performed on a training set of 39 polysomnography (PSG) and demographic variables based on Analysis of Variance with P <0.05 indicating statistical significance and a random search approach with 1000 combinations was used for machine learning hyperparameter tuning. Based on the area under the ROC curve (AUC-ROC), XGBoost was determined to be the best model for predicting presence of MSL < 8 minutes (0.71 + 0.04) and random forest for presence of ≥ 2 SOREMPs (0.75 + 0.05). By computing Shapley Additive Explanations values in a new train/test 80%/20% split sample, it was identified that the 5 most relevant variables for MSL < 8 minutes were total recording time, percentage of sleep time, wake after sleep onset, non-supine sleep time, and sleep efficiency. The 5 most relevant variables for presence of ≥ 2 SOREMPs were REM latency, age, ESS score, percentage of REM sleep, and minimum oxygen saturation. Overall, these results show that the preceding night PSG provides predictive information that can be leveraged to anticipate MSLT results at the individual level. This provides the foundation to better identify patients with central disorders of hypersomnolence based on PSG.