Predicting Gastric Cancer Risk: A New EHR-Based Model
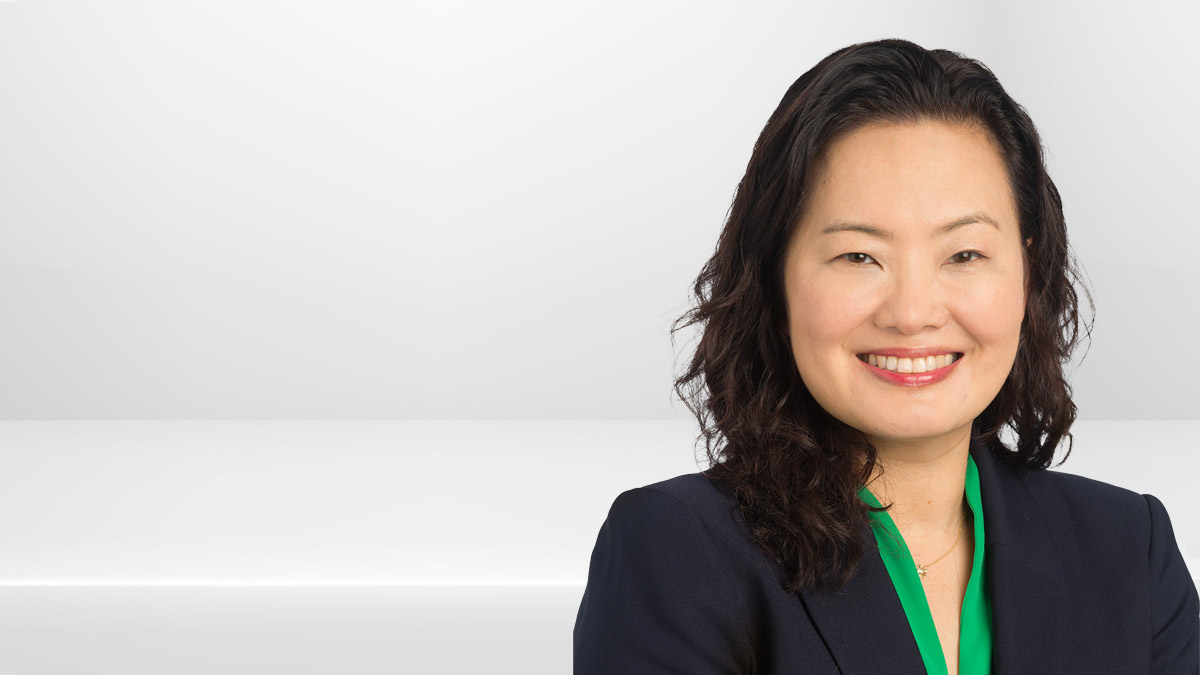
Chair of the Department of Gastroenterology, Hepatology and Nutrition, Michelle Kang Kim, MD, PhD, joins the Cancer Advances podcast to discuss her team’s innovative work using electronic health records (EHR) to develop a predictive model for noncardia gastric cancer. Listen as Dr. Kim explains how the model uses common clinical variables to flag high-risk patients who may benefit from endoscopic screening.
Subscribe: Apple Podcasts | Podcast Addict | Buzzsprout | Spotify
Predicting Gastric Cancer Risk: A New EHR-Based Model
Podcast Transcript
Dale Shepard, MD, PhD: Cancer Advances, a Cleveland Clinic podcast for medical professionals exploring the latest innovative research and clinical advances in the field of oncology.
Thank you for joining us for another episode of Cancer Advances. I'm your host, Dr. Dale Shepard, a Medical Oncologist, Director of International Programs for the Cancer Institute and Co-Director of the Sarcoma Program at Cleveland Clinic.
Today I'm happy to be joined by Dr. Michelle Kim, Chair of the Department of Gastroenterology, Hepatology and Nutrition. She was previously guest on this podcast to discuss neuroendocrine tumors and to discuss neuroendocrine tumors and carcinoid heart disease. Those episodes are still available for you to listen to. She's here today to discuss the electronic health record-based model to predict noncardia gastric cancer risk. So welcome back.
Michelle Kang Kim, MD, PhD: Thank you so much for having me.
Dale Shepard, MD, PhD: So, remind us a little bit about what you do here at Cleveland Clinic.
Michelle Kang Kim, MD, PhD: So, my day job is really, as you mentioned, chair of the Department of Gastroenterology, Hepatology and Nutrition. And in that capacity, essentially I'm responsible for the clinical operations as well as the academic mission, i.e, the research, education, and innovation of the entire department. We're very fortunate to have a staff of 100 gastroenterologists, psychologists, and other folks just in Northeast Ohio. And of course, we also have our global enterprise as well.
Dale Shepard, MD, PhD: Excellent. So we're going to talk about gastric cancer today. A lot of different people might be listening, and give us a little bit of a... just start out, let's get a broad overview, what is gastric cancer, who gets it, what's the incidence?
Michelle Kang Kim, MD, PhD: Yeah. So as you mentioned, I generally speak on neuroendocrine tumors and carcinoid tumors, and this has been a fairly recent interest of mine, really born from, I think, some information I had gotten when I realized that gastric cancer is associated with awful morbidity and mortality. And that really struck me as a tragedy as this is something that we can actually detect endoscopically early and actually has the potential for cure.
In the United States, it's not a very common cancer, probably about 7 per 100,000 similar to that of cervical cancer. But actually worldwide, it's one of the most common cancers that we see and responsible for one of the top five leading causes of death.
Dale Shepard, MD, PhD: And so, we're going to talk a little bit about maybe a prediction of who might get these gastric cancers, and of course that's important because you mentioned that if you find it early, the people could be cured. What are the barriers right now to screening? Is there screening, do we do screening in the U.S.? And if not, why not?
Michelle Kang Kim, MD, PhD: Right. So we do not screen for gastric cancer in the U.S., and that's largely born from the fact that it's not very common. I did say that it has an incidence of about 7 per 100,000, and that, of course, we do screen for other cancers like that, like cervical cancer for which the incidence is equal. The issue is that you are also screening by a different modality, that you're screening with an invasive procedure, upper endoscopy, and so that's really the reason that it's not done in the U.S.
Having said that, it is done in many other areas of the world where it's much more common. And that's really what gave me the idea that perhaps in other countries where the incidence is higher and they can screen the population at large, that's one thing. But that in the U.S., if we could identify a population that it was of high enough risk, maybe we could screen for gastric cancer in this country. And that's really what set this entire question off.
Dale Shepard, MD, PhD: And you talk about different way that we would screen for gastric cancer, maybe briefly describe what that would entail.
Michelle Kang Kim, MD, PhD: So, the classic methodology is really with some sort of imaging. So again, in East Asia in Japan and Korea, they will screen either by upper endoscopy, which is the most common modality. A number of years ago, they would also screen by upper GI series.
I think our thinking is that if there is a way to screen for gastric cancer in the U.S., it probably will be with upper endoscopy. There are no good biomarkers or any other blood tests that might identify a higher risk enough cohort. And so the idea is that we would be able to screen by upper endoscopy.
Dale Shepard, MD, PhD: Excellent. So we're going to talk a little bit about some work you did trying to identify maybe groups that are at higher risk and using the electronic medical record. Just one more thing about the cancer itself. This particular work, you focused on what's called noncardia gastric cancer. Tell us a little bit about what that means and why that was the area chosen.
Michelle Kang Kim, MD, PhD: Right. So in trying to predict something like gastric cancer, you want to identify a cohort that has similar risk factors associated with the development of that cancer. So we actually excluded a number of different cancers. Noncardia was certainly one of them. The reason being that that's really much more akin to, let's say, esophageal cancer with similar risk factors, and the risk factors do not mirror in noncardia gastric cancer, which is why we excluded those of the cardia.
Dale Shepard, MD, PhD: And then you mentioned exclusions, so things like GIST tumors and things like that.
Michelle Kang Kim, MD, PhD: Exactly. So there are a lot of other tumors you can get in the stomach that are not as common as our classic noncardia gastric adenocarcinoma. So that includes the GIST tumors, the carcinoid and neuroendocrine tumors, other types of tumors, which again, may not have the same risk factors as what we classically associate with the noncardia gastric adenocarcinomas. And so for that reason, we decided to really try to get a very homogeneous, well-characterized cohort, and we excluded all these other pathologies.
Dale Shepard, MD, PhD: So for this particular work, you use the electronic medical record. So tell us a little bit about how you set about doing this work.
Michelle Kang Kim, MD, PhD: Yeah. So it's funny how you can attend talks and listen to podcasts like this and sort of have ideas. And I remember thinking from a talk that I had attended at my previous institution that the risk factors for gastric cancer are very simple, and what seems to be very simple in what is an extremely complex advanced technology age. So these risk factors include age and gender, certain races and ethnicity, H. pylori, family history.
And again, in an era where we have personalized medicine and we have so many other genetic and other more sophisticated biomarkers, this seemed to me to be very crude and something that we could use the electronic health record very effectively for. And this is really why I decided to use this as a potential source for identifying patients.
The other thing is that with gastric cancer, we want to try to detect it early. The way that patients are presenting right now is that they're presenting late with gastrointestinal bleeding, with anemia, and these patients have stage three, stage four cancer. And while it certainly can be treated, the opportunity for detecting those cancers earlier has already been lost. And that's where I really wanted to try to get these patients earlier, identify them earlier, and perhaps then bring them in for an upper endoscopy if we deem them to be of high risk.
Dale Shepard, MD, PhD: And so when you set upon using the medical record, just logistically, tell us a little bit about what does that look like? Seems like a daunting task.
Michelle Kang Kim, MD, PhD: It is a daunting task. It's not something certainly that came naturally. Well, in one sense, I will say that there are some things that came about very naturally, which is that we use it every day. And so it's a repository of large volume data that, frankly, we're using to capture clinical encounters no matter whether you decide to or not, if you're a physician or other caregiver and you're seeing patients, you're contributing to this repository every single day. And so it's a very powerful source of data. And I think a lot of us understand it and perhaps the things that it can contribute as well as maybe the things where the data is not as accurate.
So I think intuitively we understand what is in the medical record and how it's used, but I think what we don't do as well, and I thought this could be an opportunity as well, is using all of that data to advance health and to advance science. And that's where I thought there really, again, is an opportunity that we're not leveraging and that could provide a lot more than just be a repository for our clinical encounters.
Dale Shepard, MD, PhD: And then when you think about data extraction, was this brute force, or did you use language models to sort of extract data?
Michelle Kang Kim, MD, PhD: So here at Cleveland Clinic, we're very lucky to have a robust data analytics team. And so the first order of business was really just to identify how many patients did we have and to pull those records and to assess the accuracy.
So the first thing we did was just to take about a decade of data and look for everybody who had gastric cancer. Now we used ICD-9 and 10 codes. And using that, that captured everybody, that captured all the GISTs, the carcinoids and neuroendocrine tumors as well as the cardia cancers. And so what we did afterwards then was to do, as you mentioned, natural language processing to identify and sort of further refine that cohort so that we could make sure that at the end we had a cohort that we were truly interested in and sort of not populated by other types of pathologies that we were not interested in.
Dale Shepard, MD, PhD: Sometimes when you think about the medical record, you don't always have everything for every patient that you'd like. So how do you use the information you have and kind of fill in the blanks, and how does that work?
Michelle Kang Kim, MD, PhD: Right So I would say that you're exactly right. There are certain fields where we have almost everything, and I would say that could includes things like age and gender. Race and ethnicity actually are also factors that we actually have with a great degree, I would say, of presence in the electronic health record.
Then there are other things that are a little bit trickier. Depending on sort of how detailed your physicians are, you may have records on family history or smoking or alcohol. There are, of course, a lot of other lab features like blood counts and comprehensive metabolic panels. You may have hemoglobin A1c's, and then people have different degrees of data with respect to imaging and endoscopy and et cetera, et cetera.
And so the first thing I think is just to understand your data and to understand which things have a lot of presence and which are perhaps modified by having a lot of missing data. And then there are ways to modify that. There can be something, for instance, instead of, let's say, with alcohol use, instead of saying sort of current versus former versus none, maybe it's just any alcohol use versus none. And so then we can sort of define the variables in different ways that will then sometimes give you a better accuracy of what the data actually is and what it represents.
The other thing though is that there are some factors that you're going to just have to accept are going to be absent in a lot of patients. So the most obvious example for this study was H. pylori. We were hoping that perhaps we could use it because of course, that is one of the most powerful risk factors for the development of gastric cancer. But we found that H. pylori was missing, not surprisingly, in over 98% of patients. And so you really can't overcome that degree of missingness with any type of imputation or other statistical methods. And so we decided that rather than include a variable that would have so much missingness, we decided to exclude it. And we can talk a little bit more about what that potentially means for the validity of our model.
Dale Shepard, MD, PhD: Sure. So when you sort of gather all the data, you take a look, you look at the predictors, what ended up being some of the stronger predictors?
Michelle Kang Kim, MD, PhD: Yeah, so not surprisingly, some of the ones that I mentioned did end up being strong predictors. So it's not surprising that age matters. As you get older, you have a higher risk of developing a noncardia gastric cancer. Same thing with gender. We know that occurs more in men, and so again, not surprisingly, that was a very strong risk factor.
And then I would say the next 10 to 15 risk factors were things that were surprising and things that were aligned. And so family history, certain races and ethnicities, certain types of blood tests and lab values also came back significant. And what we were struck by, again, here is the simplicity of these different variables. None of these are things that are out of reach of any patient. These are things that you can know by looking at somebody, by doing one clinical encounter with them. A blood count is something that's very common. And so I think that was very interesting to all of us that it could be very simple risk factors, diagnoses that are very common that perhaps could predict this risk.
Dale Shepard, MD, PhD: And so, I guess you mentioned some things were surprising, some things not so much. What was the biggest surprise?
Michelle Kang Kim, MD, PhD: I think there are some things that we're still trying to understand because one of the things when you develop a model like this is that it doesn't tell you that there's definitely a biology. It just tells you that there's some association, and you have to figure out what that association is. So yes, there were some risk factors like high blood pressure, hypercholesterolemia, liver disease, other things that didn't seem to have a correlation. And so while we don't quite understand the relationships between these ICD codes and diagnoses and gastric cancer, I think what it demonstrated to us was that there could be some novel factors that we just don't understand and perhaps we need to do some more work to understand them better.
Dale Shepard, MD, PhD: Were there any factors that you saw that were maybe not as much of a factor as you might have thought? So oftentimes you hear smoking is related to a lot of things, lung and bladder and gastric gets thrown in that as well. But if you look at the odds ratio is like 1.6 or something. Were there any that were maybe not quite as much of a factor as you might've anticipated?
Michelle Kang Kim, MD, PhD: I think actually we were pretty pleased that most of the variables that we thought would rise did rise, and we were not really that surprised, honestly, because I think we're understanding that even our capture of this data is relatively fluid and it can really sort of depend, again, on the data that's being inputted into the EHR. So I would say much more than the specific risk factors, it's really much more a demonstration of feasibility of using this method to identify high risk candidates and perhaps to, again, use this type of data science to be able to do this for other diseases. So it's really much more the platform, I would say, than the actual specific risk factors.
Dale Shepard, MD, PhD: Yeah. And if you look at the model in general, were you happy overall with its predictive value?
Michelle Kang Kim, MD, PhD: Honestly, we were quite happy. I think, again, going into this, we had never done this before, and there's limited data on what the EHR can do. So actually we were very pleasantly surprised that it could do this well, again, with such crude risk factors. I think we were even more surprised when we also not just developed and tested the model, but also when we validated it in another population.
So we actually developed an internally tested in Ohio data from Cleveland Clinic, and then we actually validated it in a separate population that was not used for the development with Florida data from CCF. And so the CCF Florida population is very different from the Ohio population. You have a stronger Latino presence, you have different doctors, different demographics, different practice patterns, different geography, and what was very surprising to us was actually that the performance of the model was sustained even in this external validation of a very different population. So that was very interesting and I think a very reassuring sign to us that we could be onto something.
Dale Shepard, MD, PhD: A lot of people listening in might not be remembering their statistics, and so anytime you think about these models, you think about specificity, you think about sensitivity. Give us a little bit of an idea about those characteristics for this.
Michelle Kang Kim, MD, PhD: Sure thing. So again, a model is just a way to define sort of how you're going to predict an outcome. In this case, gastric cancer risk. And so you can actually set your threshold for different levels where, for instance, if you have a very selective threshold, you won't capture many patients, but you will have a very specific model for capturing those patients. Now, that's not really ideal because you want to do more than capture one or two patients who are going to develop gastric cancer.
On the other hand, you could have a highly sensitive model, but then the specificity will be very low. And that's not great either because then you're saying, well, all of you 100 people are going to be high risk, but actually only, let's say, 30 of you are actually going to have cancer. You've just sort of subjected 70 people to a great amount of anxiety and distress about being labeled as high risk.
So it's really sort of finding that area in the middle, that sweet spot where you can optimize the accuracy of the model as well as the sensitivity and specificity and have something that is actually reasonable to implement.
I would say that in our paper, we recently did talk about the different thresholds of the different sensitivities and specificities that you could have, but at this time, I would say it's certainly premature to be thinking about implementing this. You would need much higher sensitivity, specificity, positive predictive value in order to be able to implement a test into practice.
Dale Shepard, MD, PhD: And I guess when we think about implementing a test into practice, what is that additional work that is going to be required? Is it going to be taking maybe the model and prospectively screening and seeing how successful it is? What is it going to take?
Michelle Kang Kim, MD, PhD: So it's a good question. So it's actually the subject of our NIH grant where we have a collaboration not just with Cleveland Clinic, but also with Columbia University in New York as well as the University of California, San Diego and California. And the idea being really to expand what we've done. What we've done is terrific in terms of Cleveland Clinic data, Ohio and Florida. But now we really want to expand and sort of assess the generalizability and the validity of this model. In order to do that, you have to really have data from different areas in the country to see how the model does in these different areas.
So actually the next several years will be devoted to sort of redeveloping a model and really refining a model with our data and Columbia data, and then validating it in California. And I think that if we see, which we hope we will, that perhaps a refined model, I don't think it'll be the exactly the same as what we have today, does well enough in terms of accuracy, sensitivity, and specificity. Then this now is where you can certainly validate it prospectively.
The other thing that we're proposing to do also is actually to do a simulation model, and that way you can, without sort of subjecting patients to prospective upper endoscopies for research, you could sort of simulate the model and simulate that natural history and see if we can detect, at least on simulation, the detection of gastric cancer.
Dale Shepard, MD, PhD: So it sounds like a great way to sort of develop the right cohort of people that could benefit from screening, but this is going to be a long time before that happens and we have that information.
Is this in any way, although I realize it's not validated, is it in any way something that you, your colleagues, others could say, "Well, if I have people that I'm suspicious..."? Screening, of course, being people without symptoms, I realize, but maybe a little bit higher level of suspicion looking earlier, maybe not necessarily formally screening, but using this in some way until we have that definitive answer to do the right thing perhaps by patients.
Michelle Kang Kim, MD, PhD: Right. Well, I will say that informally and anecdotally, gastroenterologists are already doing this. If you're from, let's say, a high incidence country, let's say you are from Japan, Korea, if you're from South America or you have a family history or you have anything related to H. pylori, you will likely get an upper endoscopy.
So I would say that we sometimes have to, of course, find a way to have this done and covered by insurance, which is a different matter, but we are already doing this informally.
The other thing I will say is that I do see a movement in general in gastroenterology that there potentially could be gastric cancer screening, again, for high-risk patients. There's been some work looking at cost-effective analyses, and I see there is a growing interest in a wave of interest in this field. I think especially because it is something that is curable really if found early.
So I think not necessarily because of our model, but I think more just because people see the opportunity and people see the really how awful it is to have patients diagnosed when they have metastatic disease, when there's an opportunity to diagnose them earlier, that I think that whether it's through this study or through other studies as well as our own clinical experience, I do foresee in the next 10, 15 years or so, I do think that we will start to screen for gastric cancer in certain cohorts.
Dale Shepard, MD, PhD: That's great, because as you say, it's a bad disease when they get to us as the medical oncologist. So this is fantastic that you're doing some work to try to prevent that.
Michelle Kang Kim, MD, PhD: Yeah, thank you.
Dale Shepard, MD, PhD: So, appreciate you being with us to share your insights.
Michelle Kang Kim, MD, PhD: Oh, it's my pleasure. Thank you so much.
Dale Shepard, MD, PhD: To make a direct online referral to our Cancer Institute, complete our online cancer patient referral form by visiting clevelandclinic.org/cancerpatientreferrals. You will receive confirmation once the appointment is scheduled.
This concludes this episode of Cancer Advances. For more podcast episodes, visit our website, clevelandclinic.org/canceradvancespodcast. Subscribe on Apple Podcasts, Spotify, or wherever you listen to podcasts.
Thank you for listening. Please join us again soon.
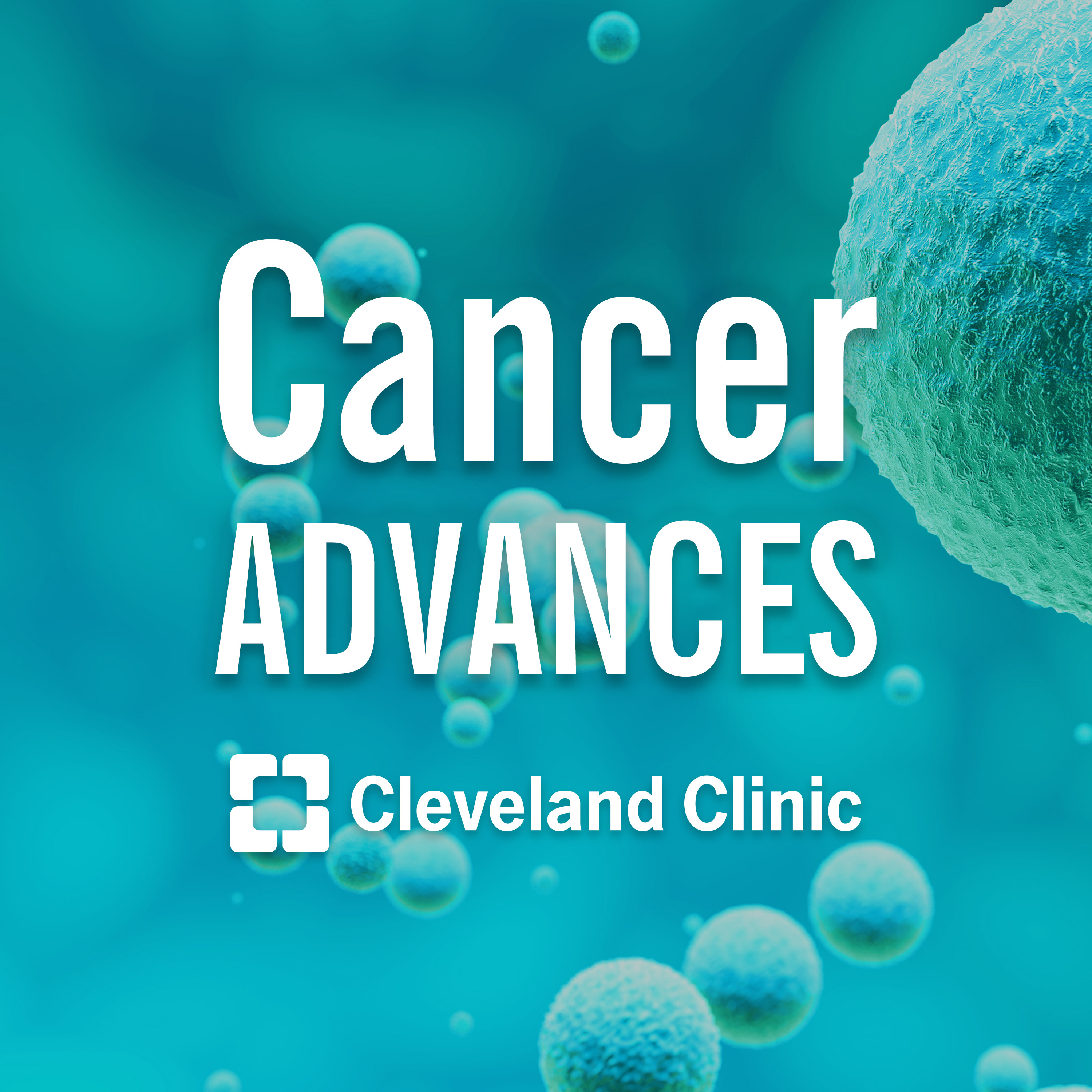