AI-Supported Mammography
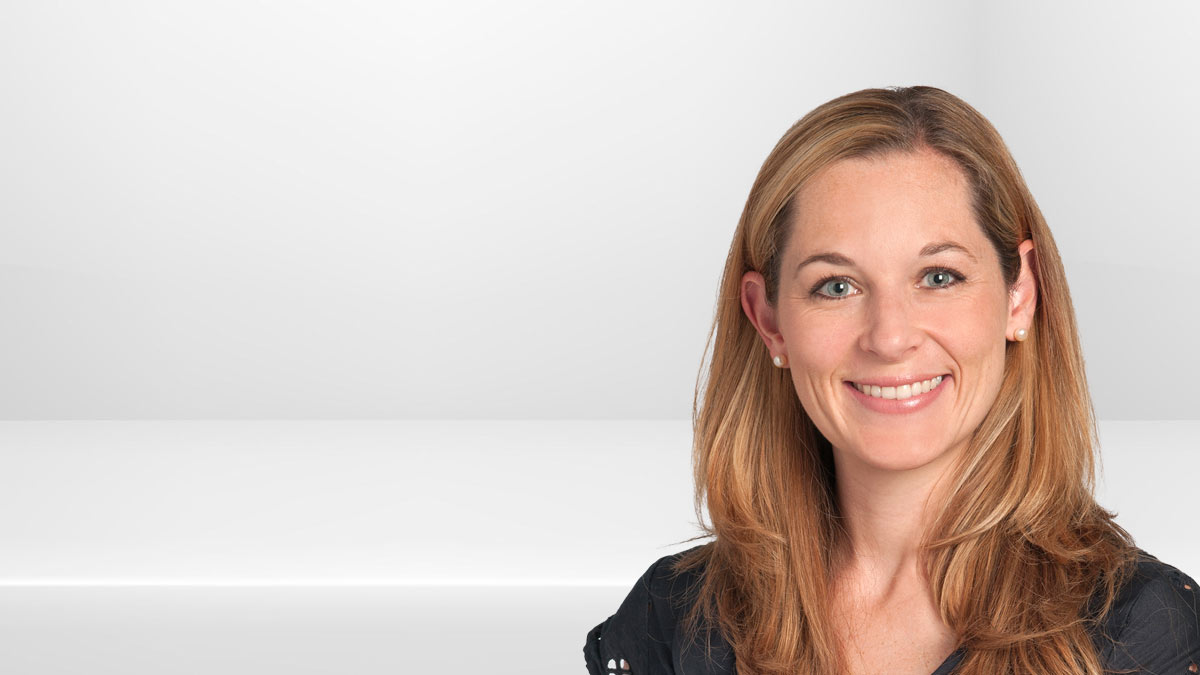
We kick off Breast Cancer Awareness Month with radiologist Laura Dean, MD, who discusses the incorporation of artificial intelligence (AI) into mammography for breast cancer screening and detection. Listen in as our host, Dr. Dale Shepard, talks with Dr. Dean about the new AI algorithms that have improved the accuracy of mammogram interpretations. Dr. Dean explains digital breast tomosynthesis (3D mammography), the benefits of AI in reducing callback rates, and more.
Subscribe: Apple Podcasts | Podcast Addict | Buzzsprout | Spotify
AI-Supported Mammography
Podcast Transcript
Dale Shepard, MD, PhD: Cancer Advances, a Cleveland Clinic podcast for medical professionals exploring the latest innovative research and clinical advances in the field of oncology. Thank you for joining us for another episode of Cancer Advances. I'm your host, Dr. Dale Shepard, a medical oncologist here at Cleveland Clinic directing the Taussig Early Cancer Therapeutics program and co-directing the Cleveland Clinic Sarcoma program. Today I'm very happy to be joined by Dr. Laura Dean, a diagnostic radiologist here at Cleveland Clinic specializing in breast imaging. She's here today to talk to us about AI-supported mammography. So welcome, Laura.
Laura Dean, MD: Hi, good afternoon. Thank you so much for having me.
Dale Shepard, MD, PhD: Absolutely. So maybe start off, let us know a little bit about what you do here at Cleveland Clinic.
Laura Dean, MD: Absolutely. So, I am a radiologist primarily specialized in breast imaging. So essentially that means that my practice encompasses all things related to breast and breast health. Of course, that's very important, the role of breast cancer screening and diagnosis and management so that's a huge part of what I do, but really anything related to breast health, including benign breast diseases, falls into the realm of breast imaging.
Dale Shepard, MD, PhD: Excellent. So as this episode is initially being posted, it is Breast Cancer Awareness Month, and so we're going to talk about AI-supported mammography. So maybe just to start, a lot of different people listen in, may or may not be familiar with a lot of these topics, maybe just start out with a big overview about how AI has been incorporated into reading mammograms or interpreting mammograms.
Laura Dean, MD: Absolutely. So, I think it's really important when we talk about the idea of computer-assisted detection or CAD, as you'll hear it commonly referred to, you kind of have to look at how CAD was initially developed in terms of its application to mammography. So, it was around 1998, so back in the late nineties, there was a lot of excitement around the initial CAD that was developed to aid an interpretation of screening mammography. And in the early 2000s, that use kind of increased and essentially the initial CAD applied to 2D mammography, and it was designed to identify suspicious or potentially suspicious findings on the mammogram. While there was initially a lot of excitement and enthusiasm for this technology, what we sort of found as we went through the early 2000s and then kind of into the mid 2010s, and this was actually published, is that rather than actually helping radiologists to be more efficient and more accurate, it was actually making them worse in their interpretations. And this was actually published.
And so, kind of over time, a lot of radiologists essentially lost faith in that technology and dismissed it altogether and so that's completely different. So, when we're talking about AI or artificial intelligence for mammography today, we're talking about a much more sophisticated algorithm. So, we now have these more complex deep learning algorithms where the machine can essentially learn from these large quantities of data sets that are available in our radiology images and it can learn these patterns of suspicious and potentially suspicious findings. And what we were finding is that it's much, much more accurate as opposed to the initial CAD that people were initially introduced to.
Dale Shepard, MD, PhD: And I guess just not really my clinical area, but just sort of thinking back in lay press and things, enthusiasm when computerized reads started, but wasn't there a little bit of apprehension as well and people saying, "Do I really want a computer reading instead of a person?"
Laura Dean, MD: Absolutely. There's always the question, certainly from the mind of the patient, "Where is the doctor?" And in radiology we're always kind of the face behind the machine anyway, so a lot of patients don't really know us as radiologists, and so we're sort of an abstract figure. So, I think that makes it a little bit harder for patients, number one, to know and trust their radiologist. And then if you add a computer learning or a CAD on top of that, is the radiologist behind the scenes even looking at my mammogram? So that's one part of it.
And then I think patients also from the radiologist standpoint, radiologists themselves weren't even trusting the technology. So how can we get our patients to really accept this if the radiologist isn't really trusting what it's telling it? And that's where we've really noticed a shift in the newer AI techniques that we're seeing within the last couple of years, it's been a huge change in the evolution of that technology.
Dale Shepard, MD, PhD: And I guess talking about technology patients, providers, they see terms and phrases and digital breast tomosynthesis, give us a little background on what exactly that is.
Laura Dean, MD: Digital breast tomosynthesis is a huge piece of this new AI puzzle. So, when you hear about digital breast tomosynthesis, which is kind of a mouthful, you'll hear that commonly referred to as a 3D mammogram or in radiology, we just call it tomo. So, what tomo is essentially that 3D mammographic picture. So as opposed to the traditional 2D mammogram where you had superimposition of breast tissue and that led to the potential to miss cancers because of a masking effect, the 3D mammogram really eliminates that overlap effect. You can almost think of it as like a mini-CAT scan for the breast. So, the radiologist has the opportunity to really scroll through that breast tissue, especially in patients who have dense breast tissue, it eliminates that overlapping breast tissue phenomenon.
So, it increases our detection of cancer in dense tissue, and it decreases the chances of a false positive such as a callback for something that was just overlapping breast tissue. So, when we talk about tomo with respect to the newer AI technologies or newer CAD, that's really where we're focusing. So, we're focusing on these newer deep learning kind of AI algorithms as they apply to cancer detection for 3D mammography or tomosynthesis.
Dale Shepard, MD, PhD: So really just being able to more effectively utilize the data that we're collecting?
Laura Dean, MD: That's absolutely right, and what these studies have been showing us when we're looking at how radiologist performance has been affected by these newer AI algorithms, and there are a number of them that are now on the market. But what the initial reader studies are showing us is that it's helping radiologists, number one, to be more efficient. So, their reading time is being cut as much as in half, especially when we're reading these tomosynthesis images where we have hundreds of images that we have to sort through for one patient. So, without the CAD to help us go through, it's double reading time if not even more for one single screening exam. So, when you're going through a whole bunch of patients over the course of the day, that's a really significant amount of time. So, it's showing that it's increasing efficiency for the radiologist by cutting their reading half in time, and it's helping them to be more accurate so decreasing the false positives, increasing the positive predictive value of a patient who's called back from mammography.
Dale Shepard, MD, PhD: Since as a radiologist, as you correctly identified, you're just kind of that person that I went in for a test and they gave me a report, practically speaking, what is a read time? How long does it take to read a film?
Laura Dean, MD: So, if we talk about a 3D mammogram, so the tomosynthesis, so you would say, so compared to a 2D mammogram, kind of the older style, just the standard four views, a radiologist, especially an experienced radiologist, could maybe read that in 15 or 20 seconds, especially if it's not a terribly complex exam. And if you compare that to a tomosynthesis exam where you're really scrolling through images in each of those four views, and so you have hundreds of images, lots of data to sort through, that could easily take two minutes if not even more. And then coupled with that, they're huge files and so sometimes they're slower to come across depending on network crowding and so forth. So, a lot of different factors which make those much, much longer and more complex to interpret.
Dale Shepard, MD, PhD: So, what has been the impact on the ability to detect breast cancers?
Laura Dean, MD: So, what we're seeing with these AI techniques is that they're helping us in a couple of ways. So, we've talked about how they are helping with efficiency. So, for radiologists who are already feeling the pressure of getting through more exams with less time to do it, that's certainly a factor helping to possibly prevent burnout. And then also they're helping to detect really, really subtle early cancers that we might not have been able to see before. So, we talk about the concept of a second reader with CAD or AI technology. And basically what that means is of course the radiologist is looking very carefully through that image dataset, but then before the radiologist signs off on that study, being able to look through the CAD and just make sure that the CAD isn't making a detection that the radiologist wasn't able to see is a really reassuring, just another set of eyes, a second look before that patient is let go for another year.
Dale Shepard, MD, PhD: When we think about mammograms and detection of breast cancer, there's this concept of interval cancers. What is an interval cancer?
Laura Dean, MD: So, an interval breast cancer is defined as a breast cancer that is diagnosed after a patient has a negative screening mammogram, so somewhere in that timeframe between one mammogram and the mammogram the next year, depending on what that patient's screening interval may be. So, in other words, a patient has a negative screening mammogram and then several months later comes in with what's typically a palpable lump, and that's usually how they're detected is the patient feels something or their doctor feels something, bringing them in for a diagnostic evaluation.
So, interval breast cancers are important because as it may seem intuitive, they tend to be more aggressive. So, this is already a mass that has gotten to a size where it's palpable by either the patient or her clinician. So, it's usually larger in size, they tend to be more aggressive, they're faster growing, they tend to have less favorable characteristics in terms of their hormone status. And so, they tend to be higher stage, they may be more likely to be lymph node positive. And so, they're really important cancers to think about and to identify, and certainly if there's a way to mitigate their development, that's absolutely critical.
Dale Shepard, MD, PhD: And what have we learned about incorporating AI in our ability to sort out those subtle differences that might lead to us minimize interval cancers?
Laura Dean, MD: What's interesting, when you look at interval cancers, there's sort of two different sets of interval cancer. So, there's the interval cancer that's a true interval cancer, meaning when you go back, even in retrospect and look at that patient's screening mammogram, there was nothing there. Either the cancer itself wasn't there or it just simply was not detectable, so that was a true negative mammogram. But on the opposite side of that, you have the sort of false negative or the so-called missed cancer where when you go back in retrospect knowing where that cancer is living in that patient's breast, when you go back in retrospect and you can see maybe there were actually subtle findings there that we could have detected. That's where AI is really helping us and that's where I've seen it in my clinical practice, those cases where what can we learn from how that area looked on the screening mammogram? Were there in fact actually signs that we could have picked up on? And I've seen multiple cases in my practice where AI is really helping us to detect those really subtle findings that we might not have seen before.
Dale Shepard, MD, PhD: It seems strictly from a patient standpoint, there's a lot of anxiety that comes with mammograms imaging, just the thought someone might have cancer and going through screening, you mentioned earlier false positive rates, things like callbacks for further imaging, things like that, certainly those huge stressors for patients. How has AI technology reading these mammograms and interpreting the data helped out with that aspect?
Laura Dean, MD: We've seen that AI is helping in terms of decreasing the callback rate for mammography. So, radiologists, as they're becoming more familiar with this technology, are able to possibly look at a finding on a mammogram, make their own interpretation, and then combine that with what AI assesses of that finding on mammography. And then they may be able to avoid a callback. If it's a finding that they think is okay and the AI is not detecting it, then it's probably okay to let that patient go. So that may be a case that we might've called that before, but now we're able to let that patient go.
So, we are seeing that it is decreasing callback rates, which is very beneficial. To that point that you mentioned, which was patient anxiety, callbacks will still happen, there's no perfect screening test. Even with our improved diagnostic tools and with the addition of AI, there are still going to be cases that we need to bring back and do further diagnostic testing on. And that's been studied, even patients who have experienced a callback on their mammogram, even though of course they feel anxiety in the moment, they do still support screening and the vast majority of them report in the literature that they would still keep coming back from a mammogram. So, it's part of it, it's something that we are very aware of. We pay very close attention to our callback rates, and we're always, always working to try and get those callback rates down.
Dale Shepard, MD, PhD: Where is this technology clinically? I mean in terms of availability, clinical use, how widespread?
Laura Dean, MD: I think it's getting more attention certainly in the media, I know at a lot of the large radiology national meetings and we're hearing about it on CNN and in the New York Times. So, I think the awareness of the technology and especially these newer CAD algorithms and these newer companies that have kind of developed this technology, I think awareness has been increasing. And so, I think it's a little bit more widespread at this point, but I think that that will continue to increase as we learn more about it and as radiologists start to see cases and examples, and as we see this in the literature where we really see evidence of people showing benefit for radiologist performance.
Dale Shepard, MD, PhD: And so, when we think about this being you go from basic mammograms and then you go to the newer tomography and then you now go to AI, what do you see as being next? What's the next step?
Laura Dean, MD: I don't think mammography is going anywhere. That has been the gold standard for screening for decades and while that technology evolves and changes and improves in terms of our sensitivity and specificity and cancer detection, I don't see that going anywhere. And what we're seeing is that AI is just even improving our diagnostic statistics even more. So, I think that that will continue to improve.
So, in addition to the cancer detection software, we are seeing AI that is working on density assessment, automated breast density assessment, which has been a hot topic in the literature for years. And so, standardized reporting of breast density is one thing that people are starting to work on. There are companies who are also working on personalized risk assessment in terms of looking at the features on their screening mammogram and predicting that patient's risk of breast cancer developing in the next couple of years. That is all still very new technology, but I think it's really interesting to think about what that might do in terms of opening the door for more personalized breast cancer screening.
Dale Shepard, MD, PhD: And so essentially using that to make predictions and maybe changing screening intervals or things like that?
Laura Dean, MD: Exactly right. Just looking from each person to person, rather looking at a huge population base with a big broad overview of screening recommendations, making it a more personalized approach to screening. And I think more will come on that as we see how technology evolves.
Dale Shepard, MD, PhD: And then I guess as we think about that personalization of screening, the question of when to start screening is always a hot topic it seems, so maybe the potential of maybe getting imaging early and being able to determine when someone needs to start a screening program perhaps?
Laura Dean, MD: I think that's a huge part of it. And we always encourage patients, certainly by the age of 30 to have a conversation with their provider, detailed risk assessment. Are they at risk of having any kind of genetic predispositions to breast cancer? Are they at high risk for other reasons? Should they start earlier or more intensive screening protocols? These are all things that we really encourage patients to discuss with their providers as there is so much controversy, as I'm sure you've seen, about when to start the screening and how frequently it should occur. And we still recommend screening mammography at the age of 40 and every year, and that's what's been studied in the literature as being the most beneficial in terms of saving lives from breast cancer but having that conversation with a provider is really important for the patient.
Dale Shepard, MD, PhD: You mentioned about having the ability to look maybe in a little more complex way at things like density. How do AI-mediated technologies fit in, where does MRI fit in?
Laura Dean, MD: So, MRI is sort of a specialized tool that we do use for a number of reasons. So, it's used in the high-risk screening realm, so we apply it to our patients who are at highest risk of breast cancer. There is increasing evidence that breast MRI is beneficial in patients at intermediate risk of breast cancer or in patients simply with dense breast tissue who may not necessarily be at high risk or high lifetime risk of breast cancer. We are already using computer-assisted detection for interpretation of MRI, and that's been present for years. We look at the enhancement patterns and the kinetic analysis of enhancing findings on breast MRI, and so kind of CAD as it applies to MRI has been present for a long time. But I do think the use of breast MRI and its application to a broader scope of patients, not just the most high-risk patients, but patients who are sort of at intermediate risk or have dense breast tissue, I think that more will come on that as the technology is more available to patients.
Dale Shepard, MD, PhD: But it seems like probably they still will be both useful, so using AI technologies with traditional imaging won't necessarily replace MRIs as a way to do an analysis?
Laura Dean, MD: That's correct. These exams, so if we talk about, again, mammography as the gold standard for screening, and then all these supplemental tools like you were mentioning breast MRI, we never will want that to replace the gold standard of mammography. So, it's used as an adjunct to screening, but its use I think will certainly continue to increase as insurance is also changing so patients have greater access to a breast MRI as a screening tool. And that's kind of been the result of legislation that's been over the last couple of years in terms of mandated reporting for patients to let them know that number one, they have dense breast tissue and then, number two, to mandate insurance coverage for supplemental screening for those patients.
Dale Shepard, MD, PhD: We've talked a lot about how to optimize screening, that only works if people get screening. How do we increase screening?
Laura Dean, MD: So, I think access is really important. At Cleveland Clinic, we've done a lot to increase access as much as we can, such as initiating walk-in appointments where patients can walk into several clinics, and there are a number throughout the region in northeast Ohio, where patients can walk in without an order, without an appointment just to walk in when it's convenient for them to get that screening. So, I think improving access is really important. I think dispelling the myths of mammography and the myths of breast cancer screening is really important. So, as we go through Breast Cancer Awareness Month, you'll see a lot of media efforts and it's really important that we answer those questions about when should screening begin, how frequently should it happen, and really kind of answering the questions to dispel those myths for patients.
Dale Shepard, MD, PhD: Fantastic, some really fascinating things going on within breast imaging that are going to make a big impact. So, thanks for being with us and your insights.
Laura Dean, MD: Thank you so much for having me.
Dale Shepard, MD, PhD: To make a direct online referral to our Taussig Cancer Institute, complete our online cancer patient referral form by visiting clevelandclinic.org/cancerpatientreferrals. You will receive confirmation once the appointment is scheduled.
This concludes this episode of Cancer Advances. You will find additional podcast episodes on our website, clevelandclinic.org/canceradvancespodcast. Subscribe to the podcast on iTunes, Google Play, Spotify, SoundCloud, or wherever you listen to podcasts. And don't forget, you can access real-time updates from Cleveland Clinic's Cancer Center experts on our Consult QD website, at consultqd.clevelandclinic.org/cancer.
Thank you for listening. Please join us again soon.
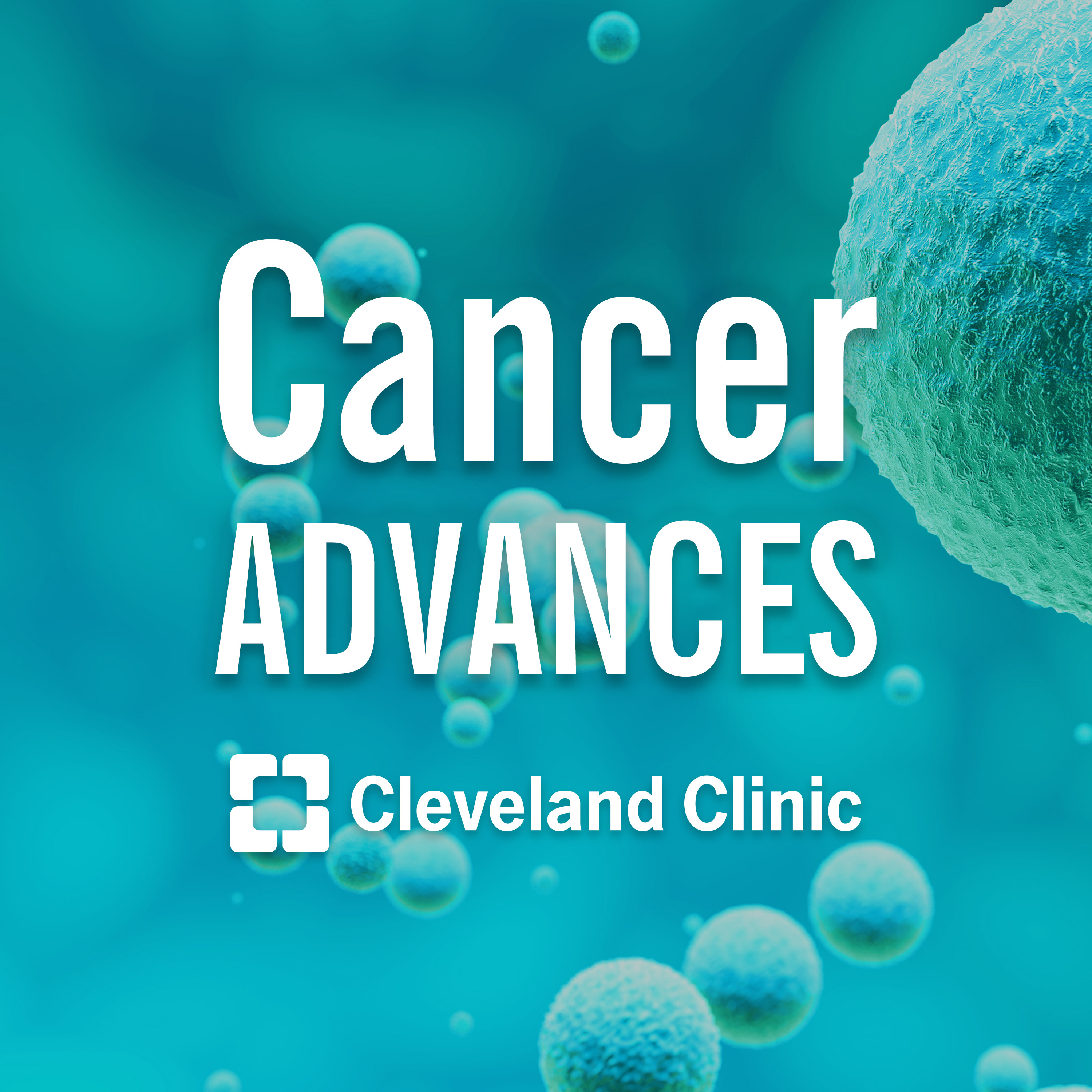