Using Artificial Intelligence to Diagnose Kidney Cancer
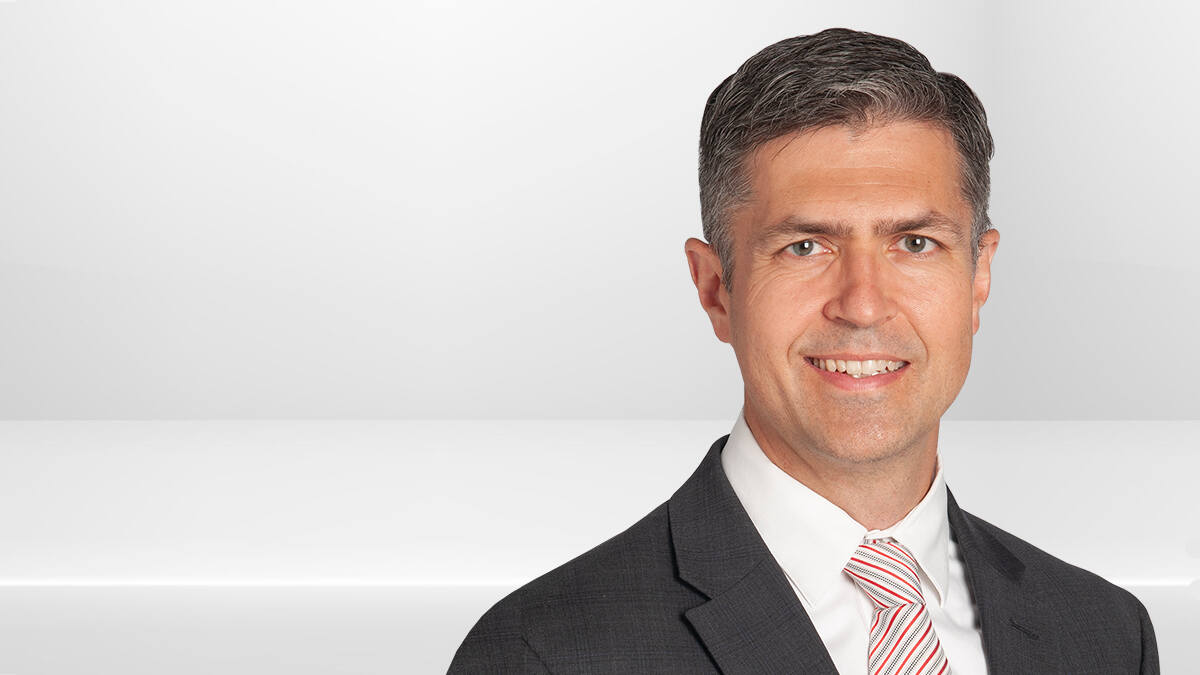
Christopher Weight, MD, Center Director of Urologic Oncology at Cleveland Clinic joins the Cancer Advances podcast to discuss using artificial intelligence to diagnose kidney cancer. Listen as Dr. Weight explains the progress we have made with imaging-generated software and a fully automated equivalent nephrometry score.
Subscribe: Apple Podcasts | Podcast Addict | Buzzsprout | Spotify
Using Artificial Intelligence to Diagnose Kidney Cancer
Podcast Transcript
Dale Shepard, MD, PhD: Cancer Advances, a Cleveland Clinic podcast for medical professionals exploring the latest innovative research and clinical advances in the field of oncology. Thank you for joining us for another episode of Cancer Advances. I'm your host, Dr. Dale Shepard, a medical oncologist here at Cleveland Clinic overseeing our Taussig Phase One and Sarcoma programs. Today, I'm happy to be joined by Dr. Christopher Weight, Director of the Center for Urologic Oncology. Dr. Weight was previously a guest on this podcast when he discussed high-intensity, focused ultrasound for localized prostate cancer and for a discussion of robot-assisted retroperitoneal lymph node dissection for patients with testicular cancer. Both of these episodes are still available. He's here today to talk to us about the use of artificial intelligence for the diagnosis of kidney cancer. Welcome back, Chris.
Christopher Weight, MD: Thanks, Dale. Great to be back.
Dale Shepard, MD, PhD: Yeah. Remind us again a little bit about what you do here at Cleveland Clinic.
Christopher Weight, MD: I oversee the Center for Urologic Oncology. The diseases that we take care of are primarily prostate cancer, bladder cancer, and kidney cancer, three of the top 10 most common cancers among men and women, and then some rare cancers like testicular cancer, adrenal cancer, penile cancer, and help make sure we offer the highest quality patient care for these kinds of cancers. Primarily, we are in the space where surgery is needed or early diagnosis and surgery, so that's where urologic oncology lives. But we work very closely with our radiation oncologist and medical oncologist colleagues as the disease progresses towards more advanced disease.
Dale Shepard, MD, PhD: All right. Well, today we're going to be talking about that early part. We're going to be talking about diagnosis and specifically kidney cancer. We're going to end up talking about this use of artificial intelligence and what you guys have been developing in that area. Give us a little bit of background though. There's a lot of different people that might be listening in with different backgrounds. What's the traditional approach to diagnosing and identifying kidney cancer?
Christopher Weight, MD: Well, most kidney cancers today are diagnosed incidentally. Or another way of saying that is that patients go in for some other complaint or concern, maybe some belly pain or maybe appendicitis or maybe they had a bad burger somewhere or something and they go in and they get a scan done and they say, wow! We may or may not see another problem, but we do see a problem brewing in the kidney. Most of them are asymptomatic and the good news is most of them are small and most of them are localized and they are diagnosed on imaging of some sort often done for another reason. It's been a good trend because if the kidney tumor is localized, it's almost always curable. A small renal mass, as we often turn them, that usually refers to a renal mass under four centimeters in size has an excellent cure rate.
We have seen really an explosion of these diagnoses. The rates have gone up tremendously over the past 10 to 20 years. But interestingly, we haven't seen an attendant drop in kidney cancer survival, at least not as big of a rise as we had seen. One of the theories was, if you were diagnosing tumors that would go on to become life-threatening and we're diagnosing them and treating them, then we should see a commensurate decline in death from kidney cancer, and we've only seen a modest decline in death from kidney cancer. What it tells us is maybe we're diagnosing a lot of kidney masses that weren't symptomatic at the get-go, but they maybe never would've become and maybe would've never become life-threatening. But the trick is, it's really hard for us to distinguish just on imaging alone between what is a life-threatening cancer and what is a cancer that very safely could be watched or observed. We're trying to understand how we really separate those two out.
Dale Shepard, MD, PhD: We talked about the imaging issues in terms of finding them and characteristics. With a lot of other solid tumors, the first thing we think about doing is getting a biopsy. Remind us about the role of biopsies in kidney cancers.
Christopher Weight, MD: Biopsy of a renal is growing in importance. It has had some shortcomings that we don't see in a lot of biopsies of other kinds of tumors, and that is that there is an inaccuracy rate that is often unacceptable to a lot of people. What I mean by that is that when you take a biopsy of a kidney tumor, there are a whole constellation of benign tumors that look like cancer on imaging and they even look like cancer or not under the microscope, and they are heterogeneous and you can't make the definitive diagnosis often without removing the entire tumor and looking through that tumor and finding the variability where you may have some parts that look not very aggressive in other parts that look more aggressive.
Biopsy definitely has a role and my gut feeling is that we will eventually get to the point where we'll be able to distinguish these much better by using a combination of biopsy and advanced imaging analysis with the use of artificial intelligence where we're going to get to a high level of accuracy that will surpass where we are right now, which is about an 85 percent to 90 percent accuracy rate, which is pretty good, but if you tell someone there's a 90 percent chance you're going to be okay, but there's still a 10 percent chance you might have a tumor there that might cause trouble, people still feel a little uncertain with that. We would like to get to a higher accuracy rate, so that we can confidently tell people this is an okay tumor to watch.
Dale Shepard, MD, PhD: Let's go ahead and jump into that whole artificial intelligence part. How did this get started? How did you get interested in this? What's the origin?
Christopher Weight, MD: Yeah. It started many years ago. Artificial intelligence is everywhere now. Unless you live in a cave, you probably have heard about ChatGPT or DALL-E 2. DALL-E 2 is an image generator where you can generate really striking artwork by putting in some descriptive terms. Of course, ChatGPT is a real-time AI where you can type in questions or prompts, and you will get surprisingly human responses. We started on this problem about five to seven years ago now, and it really started when I opened my computer one day and my photos in my photo album, my digital photos had been organized into people. They had an album of my son that I did not create, and it really freaked me out because it was pretty darn right. Every picture in the album was a picture of my son. It was over a couple of years, and he was growing and changing, and some were in profile, and some were in his track uniform, and I was like, wow.
Sometimes he was in a crowd, and I was like, what is going on here? Because I knew imaging was very important in the diagnosis of kidney cancer and we had made some efforts along with others to try to be more quantitative in how we described the tumor. By and large, the only detail that was used for 20 years of kidney cancer research and predicting how the kidney tumor would behave is just the biggest single dimensional size, the biggest diameter. About 15 years ago, there were several efforts to create some more descriptive or more quantitative ways to describe the tumor, how inside the kidney it was, how much outside of the kidney it was, whether it was in the poles or whether it was in the central part, et cetera. These systems were found to improve upon our predictions.
I started thinking, well, these computers are somehow making sense of images. They're somehow able to categorize these. I didn't understand how that could be. Imaging matters in kidney cancer description, but it's tricky to describe on a quantitative scale, five or six data points when everybody's really busy, even though it did add some statistical improvement over just size alone. I was in Minnesota at the time, and I walked across the street to our computer science department, and I said, hey, what's going on with this? How did my pictures get sorted and how can we maybe utilize this to interpret medical images? That started a fruitful collaboration that has continued over the past seven years now, and grants and papers published together with some collaborators at University of Minnesota that have been carried to here, where we're trying to take computer images of renal masses and train computers to interpret them and then make predictions based on those interpretations.
Dale Shepard, MD, PhD: It's been a long, long-term effort. How far along are we at this point?
Christopher Weight, MD: We've made a lot of progress, but we still have a long way to go. We have created a fully automated equivalent nephrometry score. This is the scoring system that I was referred to. There were several different systems where you essentially make a sub-score in five or six domains and then you quantify that and then you use that to predict outcomes. Is the tumor a cancer or not? Or is cancer a high-grade cancer or is it low-grade? Is it a high-stage or a low-stage? We have created that score and the scores that we have created using artificial intelligence have been equivalent in predicting at least those four outcomes to human-generated and expert-generated scores. That's a landmark achievement and we're really excited about that. Unfortunately, those scores, although they were better than size alone, still leave us with some inaccuracy and we're still hovering in this 80 percent to 90 percent range.
I guess what I would say is we're getting very close to replicating human expert interpretation. Now, I think we're poised to hopefully make the next jump where we can surpass human expert interpretation in predicting, especially between whether a tumor is malignant or benign or maybe even more useful, is this is a tumor that needs to be treated or a tumor that doesn't need to be treated now, and I think we're getting close, but we're not quite there yet. I think if you have seen some of these imaging-generated software like DALL-E, if you haven't gone on to DALL-E 2, you should go play around with it. It's mind-blowing.
They've made such rapid progress because images artwork is plentiful. There's no end to the number of images that you can train and "teach" the computer to understand. It's really, you're making a series of associations between how humans interpret images and how the computer program should generate an image that represents similar sort of imagery that people will interpret the same way. For their training, they had millions and millions and maybe even approaching billions of images to train. Medical imaging is much more difficult because although we have millions of medical images, these images must be annotated, described, segmented as what we often call, and then they must be able to be released to these computers to train them, and that is a logistical burden that's very difficult. It's very important to protect a patient's privacy.
There are a lot more limitations on medical images that have slowed down the advances that we've made so far, and that is another thing we're working on, is trying to help overcome these challenges where we need to maintain patient privacy, but to get millions of images to the algorithms to be able to train them, because I'm totally convinced once we do, we will see a surpassing of the interpretation of the image and that computers will be able to find and pull out details that the human eye or at least quantify them in a way that the human eye can't. Then hopefully, we'll get to that alluring point where we can really predict even better than we can right now and then it becomes very scalable. It can go anywhere in the world and hopefully democratize healthcare.
Dale Shepard, MD, PhD: I guess, when we've talked about imaging, just to clarify, are we talking simply CT imaging, PET scans, MRIs? What kind of imaging is in play here?
Christopher Weight, MD: Our initial efforts have all been focused on CT scans. That's the most common modality. It's the cheapest method. It's the most universally available that has a standard orientation, et cetera. It lends itself the best to the most used modality. There are efforts, not by us, but by others. Just recently a paper doing the same sort of thing in MRI at University of Pennsylvania that just recently came out. Clearly, it applies to all medical images.
You started some of our discussion today asking about biopsy and biopsy clearly, when you take a biopsy, you'll have a picture. You have a photo micrograph that you can also train, and that's the next step that we're working on is, can we bring in these biopsy specimens, H and E slides and combine them with the CT scans to improve upon our prediction of how patients are going to do and therefore we can personalize care. All of these types of images are being evaluated. Our research is primarily focused on CT scan and moving now towards H and E imaging. Though MRI, it's the same sort of process. It's just another matter of making the labeled dataset to train the computers on.
Dale Shepard, MD, PhD: In terms of being able to improve, you mentioned H and E slides. Are there any other markers or biomarkers or serial imaging or anything else that's being looked at this point?
Christopher Weight, MD: Yeah, that's a great question. We do have a cohort in collaboration with the Fox Chase Cancer Center of Serial Imaging, and that's a very exciting cohort for us. We do know some details. It's not like it's a blind question whether you see a renal mass and you're like, is this cancer or not? We definitely know that smaller tumors, while circumscribed tumors are lower risk. We do have a series of time points, and this is an exciting cohort because we have patients who have had scans over the years because they've been watched, and their tumors have not been removed. We're working right now to develop; can we predict which ones will grow and go on to need treatment and which ones will stay stable? We have replicated the size measurements. That's where we are in that project. We know size change as a predicting factor, and we have been able to measure the size.
That's something that we can do very well in an automated way. We can find the kidney and we can find the tumor and size is a very easy replicable modality that we can measure, and those have correlated with the human measurements. One thing that computers can do that the human brain and the human eye can't is quantify the distribution of the pixels. What I mean by that is, each pixel has a relative brightness, and it can be quantified. You can put a number on how bright or how dark it is. Then you can also use other mathematical terms to describe, well, are they mostly bright or are they mostly dark? Those are the terms we use, which are sort of general terms, but you can put solid numbers on those. We also developed a new descriptive technique of the interface between the tumor and the kidney that you can quantify it to an exact number.
You can say, what's the differential derivative of the brightness across that boundary? You can take the average differential and you can create a very reliable number that will be the same every time, and you can use that to make an estimate of how infiltrative the tumor is. Whether this tumor is growing into the kidney with a lot of fingers or whether it's a really sharp demarcated edge and that has been correlated with outcomes as well.
We have advances that we're making there where we hope that we can describe on this mathematical level what that tumor is like, and there's some evidence that these mathematical descriptions of the tumor will offer us insight, for example, in the series that you just asked about of how that tumor might grow over time. If we know it's likely to grow, then we can take it out early and very little risk. If we know it's unlikely to grow, then we have a lot of confidence we can reassure that patient, let's just watch this tumor, the probability's low that this is ever going to cause you trouble and you can avoid an unnecessary or costly surgery and recovery.
Dale Shepard, MD, PhD: At the very beginning, you talked about small renal masses, you listed four centimeters as a size related to that. Do you see this technology being ideal for a particular size of tumor? What's the sweet spot for tumors that this would be most useful for?
Christopher Weight, MD: Yeah. The smaller tumors are easier for the algorithms to find and identify. The kidney, fortunately for an AI problem, the kidney has some advantages, meaning AI has a very high probability of helping us because the kidney is surrounded in a layer of fat, and fat gives a different signal intensity from the surrounding tissue, the kidney and the tumor, and then the nearby organs and muscles. It's this buffer zone that makes it easy for the pattern to be recognized, this is the kidney. The small renal masses also fall into an easier pattern of recognition area because they don't distort the kidney anatomy that much. When you get very large kidney tumors, they can distort the anatomy tremendously growing into other organs, disrupting other organs. They're even notorious for sending a thrombus growing into the renal vessels and then into the vena cava and sometimes even to the heart.
Those are much harder for the computer to understand because they're less common, but also because they distort the prevailing patterns. Those patterns are so markedly associated with disease that there's not a diagnostic dilemma there. We don't see that kind of pattern with a benign tumor, so when we see that, we know it is a cancer. Fortunately, I think it does segment and identify these smaller tumors well, and I think that's the sweet spot because that's where we have the most dilemma in making the diagnosis as to what to do.
Dale Shepard, MD, PhD: Makes sense. You mentioned before about issues related to access, and quite honestly, it's fantastic that you already are at a point where you match human expert radiologists because if you were able to provide that to people in the community in rural areas that don't have access to experts, that's a win. How good do you think we can ultimately get, and logistically, what does this take to make it more widely available?
Christopher Weight, MD: Logistically, I think one of the interesting things right now with technology is the technology capability sometimes surpasses the logistics of deploying it. We have the algorithm available; we're working on making a web-based interface right now, and it turns out to be logistically very complicated because it relies on a patient getting a scan, having a way to get that to us, having it be the right kind of scan and have the right phase uploaded. A lot of patients don't distinguish between MRIs and CT scans and PET scans, and they may not know. A lot of patients don't know what the scan is actually of. They know it's an MRI, but it may be of their shoulder. We've had that happen before. That's a tricky area, so we're working on that.
Then there also remains this medical legal and HIPAA-related piece. We want to be sensitive to protecting patient's privacy, but at the same time being able to manipulate and move these images around to computers that can understand them. That's another logistical and legal piece that is more complicated than the technical piece, which we have already developed. We could deploy it on whose ever scan. Then there remain other technical pieces. These types of algorithms are very computing-heavy and require some pretty significant computing resources and time.
The average scan takes about 10 minutes to run through and then interpret it and spit out, and that's fast, but that requires some computing resources to be able to do that. If you scale that to make this available to the public and then suddenly thousands of people are requesting that, that can really turn into a heavy computing burden. The logistics remains actually more complicated than the technical piece at this point, but I don't have any doubt we'll be able to overcome that at some point because computing power continues to increase.
We have a really exciting collaboration here with IBM as a part of a discovery accelerator, and I think that relationship will continue to help us to develop these technical hurdles to get to the point where we can really help patients anywhere. My goal is if you get a scan, you could have a high level read if you have a renal mass and you can get a very high level read of what that renal mass might mean because in the end, I think a lot of people will be reassured that they can probably watch their mass and not need it to be treated, at least not in the immediate future, and I think that would be a big win because we have evidence now that probably over a hundred million of healthcare expenditures are spent on benign renal masses that turn out to be benign after we take them out. Even a small cut into that can make a difference where we could maybe realign those healthcare dollars to make them more valuable.
Dale Shepard, MD, PhD: Well, you're doing fascinating work that's going to really impact patients, and we appreciate your insights. Thanks for being with us.
Christopher Weight, MD: Thanks, Dale. It is always a pleasure to talk with you.
Dale Shepard, MD, PhD: To make a direct online referral to our Taussig Cancer Institute, complete our online cancer patient referral form by visiting clevelandclinic.org/cancerpatientreferrals. You'll receive confirmation once the appointment is scheduled.
This concludes this episode of Cancer Advances. You'll find additional podcast episodes on our website, clevelandclinic.org/canceradvancespodcast. Subscribe to the podcast on iTunes, Google Play, Spotify, SoundCloud, or wherever you listen to podcasts. And don't forget, you can access real time updates from Cleveland Clinic's Cancer Center experts on our Consult QD website, at consultqd.clevelandclinic.org/cancer.
Thank you for listening. Please join us again soon.
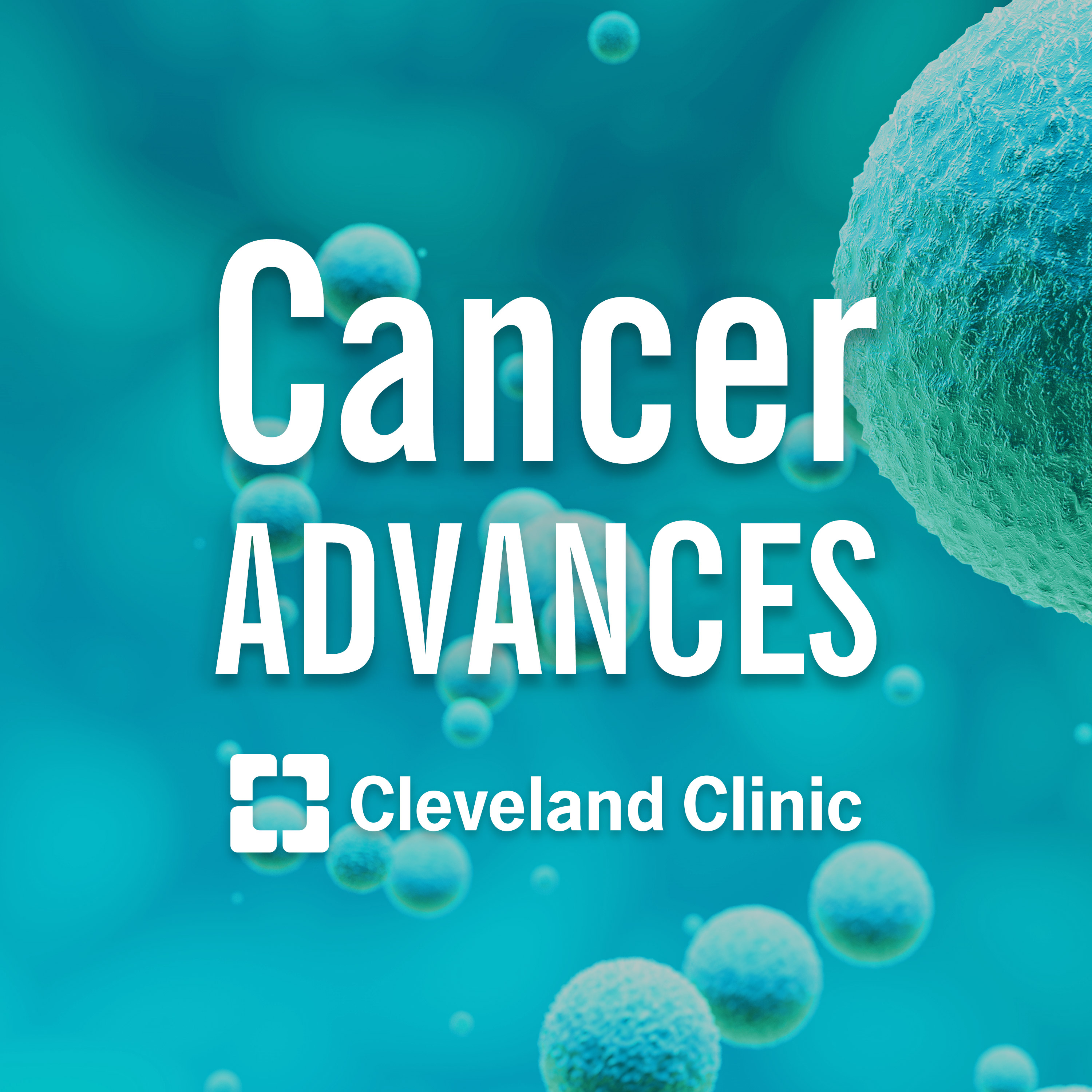